Reza Shokri
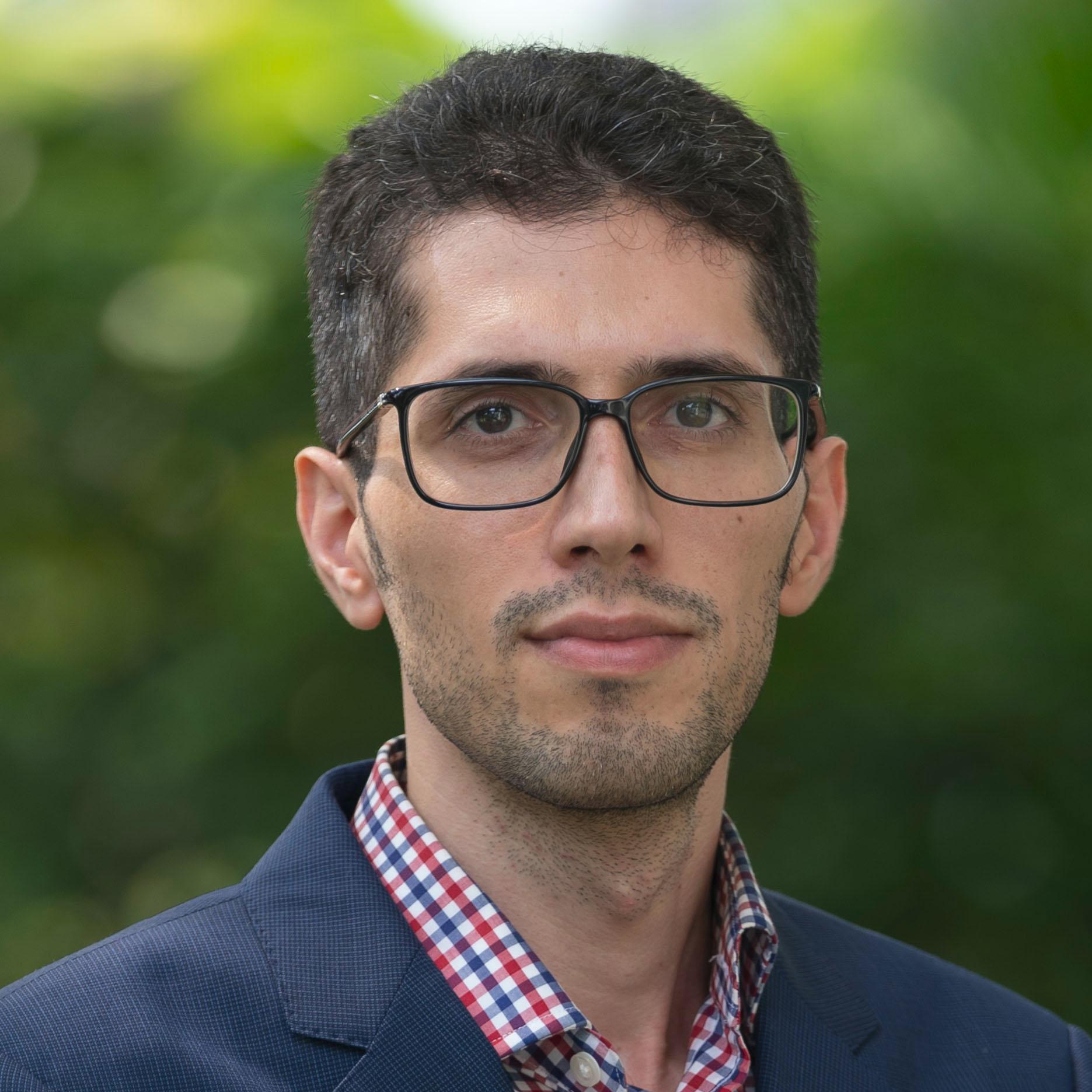
Reza Shokri
NUS University of Singapore
Reza Shokri is an NUS Presidential Young Professor of Computer Science. He received a Ph.D. in computer and communication science from the EPFL. His research interests focus on designing methods to quantitatively measure the privacy risks of data processing algorithms, and build scalable schemes for generalizable machine learning models that are also privacy-preserving, robust, interpretable, and fair.
Keynote abstract - Memorization in Machine Learning
In this talk, I will present leave-one-out distinguishability as a novel approach to measuring data memorization in machine learning models. This technique quantifies how much a model’s predictions depend on the inclusion or exclusion of specific training data points, offering a clear indicator of memorization. I will also discuss state-of-the-art membership inference attacks, which are statistical tests designed to evaluate the degree to which a model may leak information about its memorized training data. These attacks help expose potential privacy risks, demonstrating how adversaries could infer whether certain data points were part of the model’s training set.